In the fast & digitally evolving world today, staying ahead of the curve is essential, and that's where modern DevOps meets the game-changing realm of AIOps! Imagine a world where automation and artificial intelligence work hand in hand to supercharge your operations —it's not just a dream!
With AIOps adoption skyrocketing, a recent study shows that 60% of organizations have embraced these innovative tools, and the AI market in IT is expected to hit a whopping 1339.1 billion by 2030. Buckle up as we explore how the modernization of DevOps, infused with AIOps, can pave the way for a brighter, more efficient future for IT businesses!
In this blog you’ll delve into the overview of AIOps, how AIOps is important for businesses, types, differences in DevOps & AIOps, benefits of integrating AIOps in DevOps, challenges with AIs modernisation along with few great success stories slaying at their business through AIOps adoption and a checklist to check whether you AIOps is performing at its best or not! Ready to take charge? Let’s go.
AIOps Overview
Artificial Intelligence is a revolutionary force for innovation in this era. The term AIOps was first coined by Gartner in 2016. You can easily de-abbreviate it as ‘Artificial Intelligence for IT Operations’.
Though not just AI, this approach is also about utilizing all the superpowers of new-age technologies such as big data analytics, Natural Language Processing (NLP), and Machine Learning (ML) to automate identifying & resolving common IT operation issues.
Based on the Global CIO Point of View, nearly 90% of CIOs are already using machine learning in some capacity. Whereas, 87% of CIOs reported that machine learning provided “substantial value” or “transformative value” to the accuracy of decision-making. Indeed, this technology is here to stay for long!
Three stages of AIOps processing
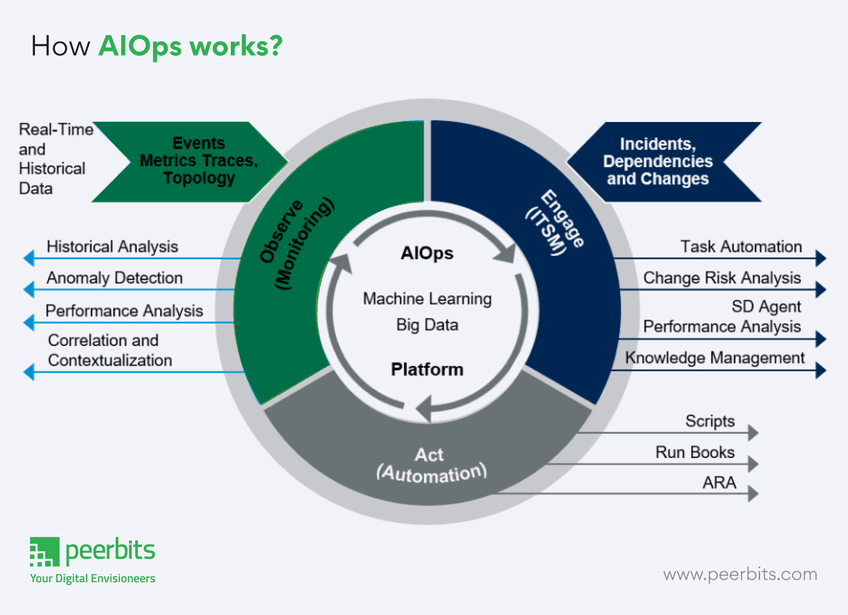
The construct of AIOps works by collecting large volumes of data from different systems and identifying the root cause of the issue for an accurate diagnosis & solution.
By utilizing big data analytics, machine learning & other technologies to their maximum potential, the working of AIOps involves 3 major stages as follows:
- Observe: It is the initial phase of AIOps. In this, AI has to identify and report IT incidents as they occur or have happened in the past. Some of its core processes are:
-
Historical analysis
-
Performance analysis
-
Finding core issues
-
Finding the overloaded devices
-
Service faults detection
-
Analyze various events, logs, and metrics
- Engage: This step is about checking on potential IT incidents and reporting them in advance. Some of its core stages include:
-
Anomaly detection
-
Changes in impacts
-
Capacity management
-
Predicting faults, overloads, or other failures in advance.
- Act: Since all the issues have been churned out, it's time for AIs to automatically fix them or share their reports with humans for final resolution. Its major stages include:
-
Root cause analysis
-
Automated or assisted maintenance
-
Automated or assisted optimization of the network.
-
Offering technical support.
Apart from AIOps, it is also denoted as - IT operations analytics (ITOA), advanced operational analytics, AI for ITOM, IT data analytics, and Cognitive Operations in the DevOps industry.
Also read- Ultimate to DevOps– principles, how it works, and real-life examples
How AIOps is important for IT businesses?
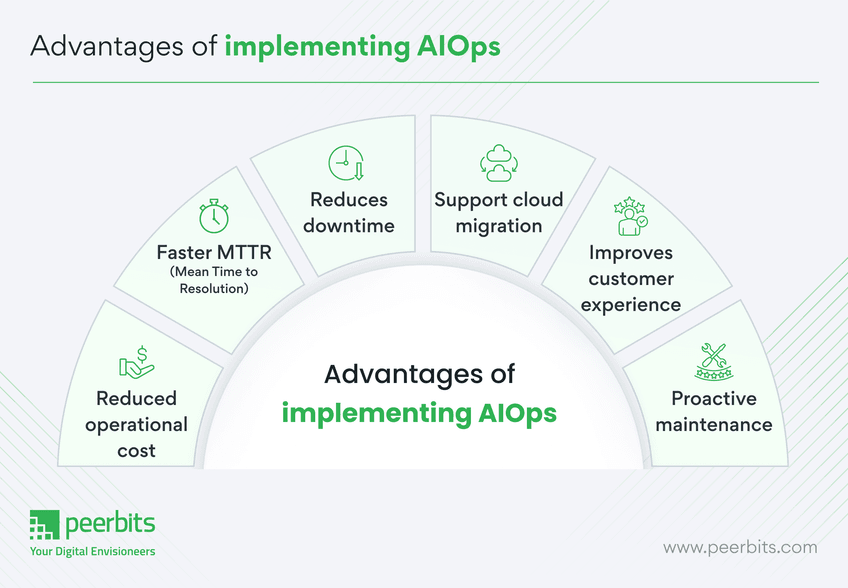
So far, you’ve understood the AIOps definition & its core functionality. But how implementing AIOps can transform your IT business? Here’s your answer:
1. Reduced operational cost
The AIOps technologies easily fetch actionable insights out of large volumes of data that required big IT teams before. This optimization of resources with a single system and lean team of data experts significantly impacts the entire operational costs. That too without compromising the functionality of IT operations. Isn’t that a great heads-up?
2. Faster MTTR (Mean Time to Resolution):
Artificial intelligence can easily function beyond the operational noise and provide faster analysis & resolution of issues as compared to manual speed, which eventually results in reduced and faster MTTR.
3. Reduces downtime:
AIOps system, not only assesses large volumes of data but also gives you a heads up for issues before they even impact operations. This significantly affects your IT system performance & helps you to be prepared for disruptions in advance. Resolving all such problems helps you avoid frequent downtimes.
4. Support for cloud migration:
AIOps platform offers an in-depth solution for managing public, private, or hybrid cloud environments. With this approach, your organization can shift workloads from traditional systems to cloud infrastructure without the complexities of intricate data transfers across the network. It enhances visibility, allowing your IT teams to efficiently manage data across various storage solutions, networks, and applications.
5. Enhanced customer experience
When AIOps resolves the majority of IT operations in seconds and identifies potential bottlenecks through extensive big data analysis, your company will naturally deliver improved service quality, becoming a signature of your brand and customer experience.
6. Proactive maintenance
AIOps technologies aids in forecasting potential issues and implementing preventive measures proactively. This means you can say goodbye to the unexpected maintenance costs that typically arise at the end of each quarter!
Difference between AIOps & DevOps
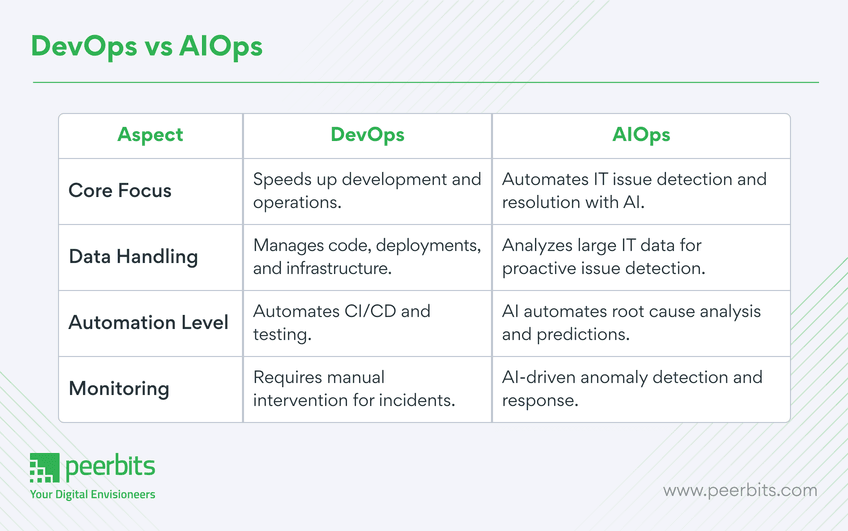
DevOps is a software practice that bridges the gap between development and support workflows. It helps organizations apply changes and quickly address users' concerns by sharing information between software and operations teams.
On the other hand, AIOps is an approach for using AI technologies to support existing IT processes. DevOps teams use AIOps tools to assess coding quality and reduce software delivery time continuously.
Whereas DevOps focuses on accelerating and refining software development and deployment, AIOps uses AI to optimize the performance of enterprise IT environments, ensuring systems run smoothly and efficiently. AIOps platforms use ML and big data analytics to analyze vast amounts of operational data to help IT teams detect and address issues proactively.
When used in tandem, AIOps, and DevOps services can help businesses create a complementary, and detailed approach to managing the entire software lifecycle.
What are the types of AIOps?
AIOps presents new opportunities for your organization to enhance operations and lower costs. However, to make informed decisions, it's essential to identify your specific needs to determine which AIOps solutions will best meet them. Here are the two primary types of AIOps you should be familiar with:
-
Domain-centric AIOps are AI-driven tools customized to operate within a defined scope. For instance, operational teams utilize domain-centric AIOps platforms to oversee the performance of networks, applications, and cloud services.
-
Domain-agnostic AIOps are solutions that allow IT teams to expand predictive analytics and AI automation beyond network and organizational boundaries. These platforms aggregate event data from various sources and correlate it to generate valuable business insights.
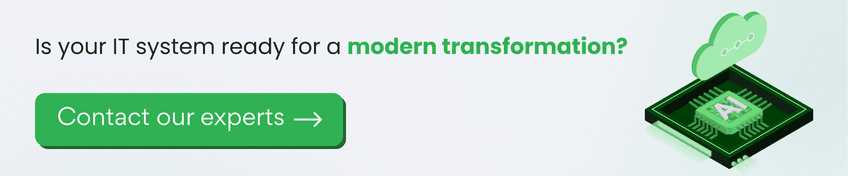
Benefits of integrating AIOps in DevOps
AIOps & DevOps both are an integral part of revolutionizing the modern-day businesses. But the significant impact AIOps implementation in DevOps brings, are as follows:
-
Increased speed and collaboration: The integration of automation enables Site Reliability Engineers (SREs) and developers to enhance their collaboration and efficiency. It results in significant savings in time, money, and resources for organizations.
-
Efficient development to production workflow: AIOps system facilitates a smooth transition from development to production for DevOps teams by automatically responding to changes within production environments.
-
Machine learning advantages: Machine learning enhances AIOps by- Identifying anomalies, anticipating performance issues, diagnosing root causes and providing insights for DevOps teams to prioritize workflow improvements and make informed decisions.
-
Consistent release-ready code: AIOps creates a framework where code is always prepared for deployment, ensuring readiness at all times.
Case studies showing successful AIOps adoption
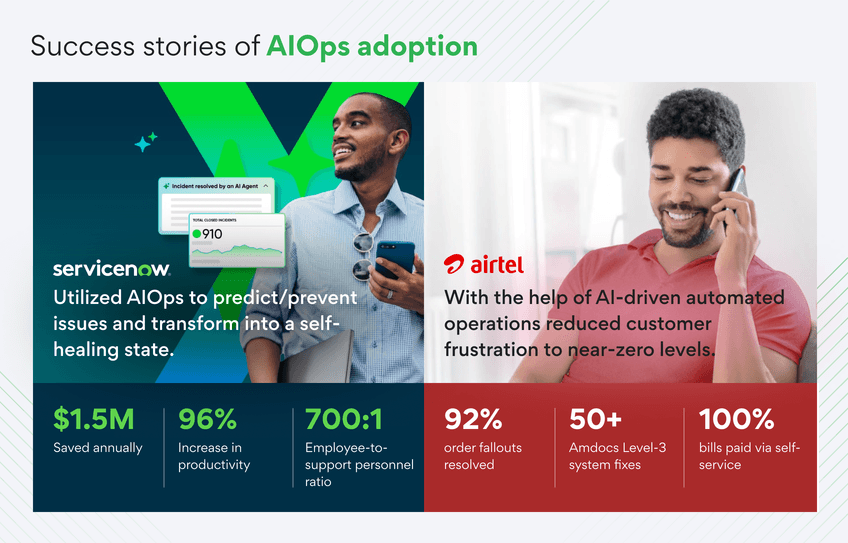
1. ServiceNow IT operation’s growth
ServiceNow has experienced rapid growth, which posed challenges for their IT operations team in consistently delivering outstanding experiences for both support teams and users. As their workforce expanded, ServiceNow found it increasingly difficult to manage the surge in requests.
They primarily focused on addressing immediate issues and responding to rising demands, struggling to onboard new talent. This led to dissatisfaction due to the high number of outages they faced. To tackle these challenges, they sought a scalable and cost-effective IT operations model and turned to AIOps.
The results have been impressive. Across the company, they have saved $1.5 million annually, improved software reclamation by 10%, and reduced events to alerts by 96%. Their employees are delighted, as reflected in their employee satisfaction score of over 99% and nearly doubled their productivity.
2. Airtel's AI-driven operations
Airtel has made significant strides in reducing customer frustration to nearly zero levels through AIOps uses in operations. By implementing an AIOps (AI for IT ops) framework and advanced automation, Airtel has increased both efficiency and speed.
AI-driven automated operations introduced a unique Customer Frustration Index to Airtel’s existing business support systems (BSS) led to the creation of allowing real-time measurement of experience improvements.
In terms of order processing they reached to 90% reduction in order fallouts, with 92% of order fallouts resolved using automated and self-healing capabilities. For bill delivery, 99% of bills were delivered within 24 hours, and 100% of bills were paid via self-service online or mobile apps accurately.
These transformations illustrate the powerful impact that AIOps system can have on IT operations and customer satisfaction. Want your systems to dope the charts too? Check our modern custom DevOps solutions.
AIOps Challenges
Surely with every new tech, there are some unexplored hazards that can limit your development growth. Out of these, the three main challenges with AIOps are skills gaps, security, and scalability. Let’s take a brief look at each one.
Challenge 1: Skills gap
Many IT departments are still in the early stages of adopting AIOps. It’s essential to invest in training and development programs to close the skills gap within your IT teams. Additionally, consider partnering with external training organizations or recruiting AIOps specialists to enhance your team's expertise.
Challenge 2: Security
The integration of AIOps tools can potentially create new security risks for your systems. It is crucial to prioritize cybersecurity measures and verify that AIOps tools meet industry standards and regulations. Implement strong encryption and access controls to protect sensitive information.
Challenge 3: Scalability
As your organization expands its use of AIOps-based systems, scalability may become a concern. Ensure that you select AIOps tools capable of growing alongside your organization. Regularly evaluate your infrastructure requirements and upgrade your tools as needed to maintain scalability.
AIOps Checklist: Assess yours now!
Make sure your AIOps is functioning at its peak with the following checklist:
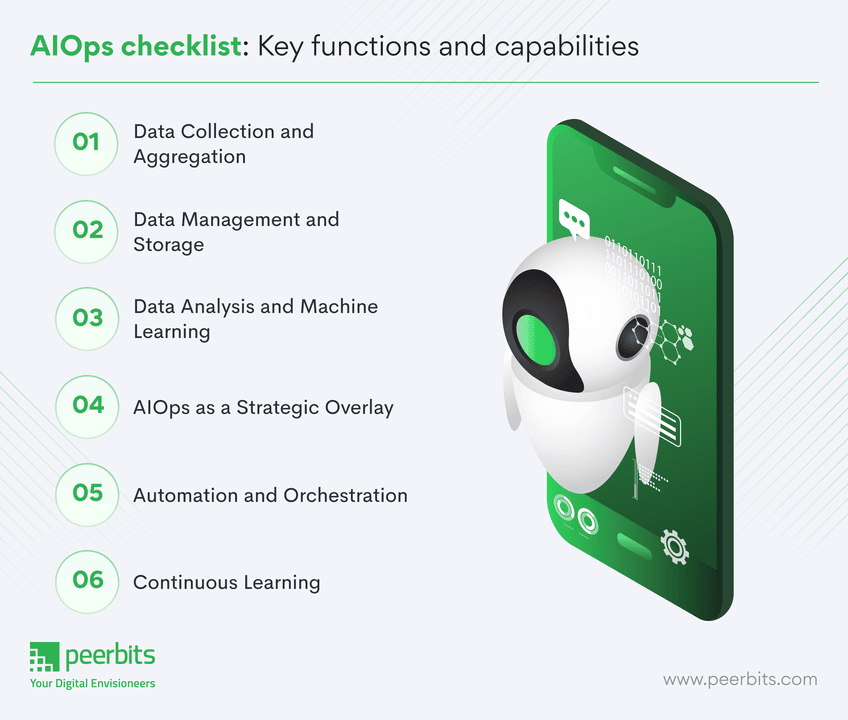
1. Data collection & aggregation
Diverse data sources: Gathers information from a variety of sources, including networks, applications, databases, tools, and cloud environments.
Various data formats: Collects data in multiple formats such as metrics, events, incidents, changes, topology, log files, configuration data, KPIs, streaming data, and unstructured data.
2. Data management & storage
Centralized access: Organize data in a central location for easier access and analysis.
Efficient management: Implement indexing and expiration processes to manage data effectively.
3. Data analysis & ML
Machine learning utilization: Use machine learning for detecting patterns, identifying anomalies, and conducting predictive analytics.
Alert significance: Differentiate meaningful alerts from irrelevant noise. Correlate and contextualize data for precise problem identification.
4. AIOps as a strategic overlay
Integration with existing tools: Combines AIOps with current monitoring tools and investments to provide a cohesive view of IT operations.
5. Automation and orchestration
Automated workflows: Transforms knowledge into automated response and remediation processes.
6. Continuous learning
Historical data utilization: Uses past data to enhance problem management and resolution over time.
Conclusion
AIOps has significant potential to transform IT operations. With the increasing prevalence of predictive analytics, automated root cause analysis, and other advanced features, AIOps is set to change how IT professionals manage and enhance complex infrastructures. Adopting these innovations and keeping up with the latest AIOps trends will be essential for maintaining competitiveness and achieving success in the ever-evolving IT operations landscape.
If you want to maximize the benefits of AI technologies like AIOps or DevOps, Peerbits can be your ideal partner for success. We assist you in automating the tracking, monitoring, and analysis of your infrastructure using best practices from DevOps and other innovative solutions, allowing you to address issues before they escalate. Staying updated is the key to today’s success and Peerbits can help you do that with ease.
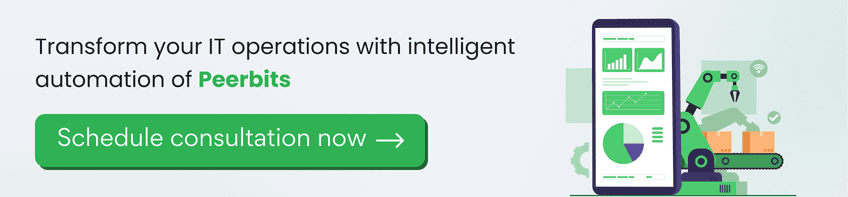